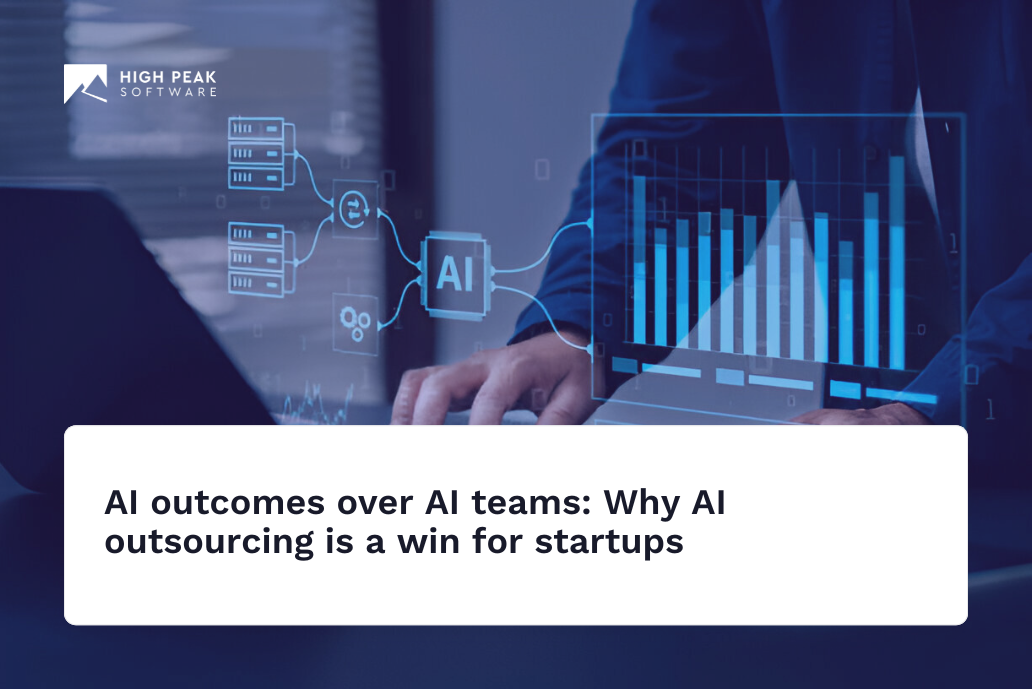
Table of Contents
- What is AI outsourcing?
- Why businesses go for AI outsourcing
- Why smart teams choose AI outsourcing: 7 benefits that matter
- The AI execution gap: Why most companies struggle to deliver results
- Why leading teams choose AI outsourcing
- Outsourcing AI strategy: How great partners lead from day one
- The hiring trap: Why in-house AI teams fail early-stage or legacy orgs
- What you avoid by outsourcing AI product development instead of hiring too early
- What you actually need: AI outcomes, not headcount
- Why AI outsourcing works in 2025
- What to look for in an AI outsourcing company
- What you actually need: a fractional AI product team
- How High Peak ships AI products
- Want to ship AI without the overhead? Choose High Peak
Everyone’s racing to build in-house AI teams. But is that really the smartest path? In a recent McKinsey survey, 92% of companies said they plan to increase their AI investments by 2028. But most aren’t asking the more important question: how will they turn that investment into actual outcomes? For many startups and mid-sized enterprises, the answer isn’t hiring—it’s AI outsourcing.
Whether you’re launching a new AI-driven feature or modernizing legacy workflows, the goal isn’t to grow a data science team—it’s to deliver impact fast. That’s why more companies are choosing AI outsourcing companies to accelerate time-to-market, reduce overhead, and stay focused on core priorities.
In this blog, you’ll learn:
- Why AI outsourcing beats in-house hiring for agility and cost-efficiency
- What top AI outsourcing companies actually offer
- Why is outsourcing AI development services important for startups
- A framework to decide if outsourcing aligns with your product or transformation goals
If you’re measured by outcomes—not headcount—this is the guide you’ve been looking for.
What is AI outsourcing?
AI outsourcing is the practice of hiring third-party companies to plan, build, and manage artificial intelligence solutions—without maintaining an in-house AI team.
These AI services can include:
- Designing and training machine learning models
- Implementing AI features into products or operations
- Managing AI infrastructure, pipelines, and performance
Startups and enterprises use AI outsourcing to reduce costs, accelerate timelines, and gain access to specialized expertise they don’t have internally. It’s a lean, outcome-driven way to adopt AI without the complexity of building everything from scratch.
Want to know about High Peak’s AI services suite? Explore High Peak’s: AI strategy consulting AI product development AI UI/UX design AI marketing |
Why businesses go for AI outsourcing
As AI adoption surges, both startups and enterprises are realizing that outcomes—not internal headcount—are what matter most. Here’s why AI outsourcing is on the rise:
- On-demand access to AI expertise: Outsourcing connects you to skilled AI engineers, domain specialists, and product-oriented technologists—without the months-long hiring timeline. Most top AI outsourcing companies like High Peak Software also bring tools and frameworks you don’t need to reinvent.
- Lower overhead, smarter spend: Building internal AI capabilities is expensive. Outsourcing sidesteps the cost of salaries, infrastructure, and training. Thus making it ideal for lean startups and transformation-focused teams with fixed budgets.
- Accelerated delivery cycles: Outsourced AI teams often work in sprint-based models, helping you move from concept to production in a fraction of the time. This is key for startups chasing traction or enterprises modernizing legacy stacks.
- Staying focused on what matters: Instead of draining resources into complex ML pipelines, outsourcing lets your internal team stay focused on core growth, customer experience, or operations. All while experts handle the heavy lifting in the background.
Why smart teams choose AI outsourcing: 7 benefits that matter
AI outsourcing is more than a shortcut—it’s a smarter way to build, launch, and scale with less risk. Here’s why startups and mid-sized companies are choosing this route in 2025:
- Launch faster: Outsourced teams are already trained, aligned, and equipped to build. You skip the hiring phase and go straight to results.
- Get end-to-end expertise: Great AI isn’t just about code—it’s about user experience, data quality, and performance at scale. The right partner brings all of it.
- Spend less, get more: You only pay for what you need—no long-term salaries, no overhead, no infrastructure to maintain.
- Build with people who’ve done it before: Top AI outsourcing companies have solved similar problems for others in your space. That pattern knowledge helps avoid mistakes you haven’t seen yet.
- Avoid dead-end prototypes: In-house teams often get stuck building proofs of concept that never launch. Outsourcing partners focus on shipping real, usable products—not just demos.
- Integrate with what you already have: Instead of starting from scratch, outsourced teams can build AI into your existing systems—saving months of rework.
- Test ideas without committing full budget: You don’t need millions to try something meaningful. A good partner helps you test and learn quickly—so you can scale only what works.
Ready to move faster with less risk? You don’t need to hire an AI team to start building. With High Peak, you get a fractional AI product team that’s ready to deliver real outcomes. Book your AI consultation now! |
The AI execution gap: Why most companies struggle to deliver results
Interest in AI is exploding—but delivery often falls flat. Startups burn runway with little to show. Mid-sized enterprises stall in proof-of-concept mode. The issue isn’t ambition. It’s execution.
- Slow execution caused by skill and alignment gaps: Most teams either hire too slowly or build the wrong team. Without product-savvy AI experts or aligned leadership, timelines break down before development begins.
- Prototypes that never reach production: Internal teams often build disconnected models with no feedback loops, UX integration, or real-world usability. The result? AI that works in a demo—but fails in operations.
- High costs, low outcomes: Recruiting talent, managing infrastructure, running experiments—it adds up fast. For startups, it’s wasted burn. For enterprises, it’s budget with no ROI.
- Missed windows for real AI advantage: The true cost isn’t just sunk time or capital—it’s what doesn’t get built: AI-powered features, workflow automation, or competitive positioning that others beat you to.
Why leading teams choose AI outsourcing
Smart companies aren’t chasing headcount—they’re chasing outcomes. Here’s what AI outsourcing unlocks that internal teams often can’t deliver:
- Clear AI strategy without internal drag: Experienced AI partners bring proven frameworks for opportunity mapping, use-case prioritization, and phased execution—without months of internal debate.
- Technical depth that’s ready to ship: Top-tier partners bring cross-functional expertise in data science, ML, deep learning, NLP, and computer vision—production-ready, not academic.
- Custom builds that align with your product, not just your data: No one-size-fits-all LLM wrappers. You get domain-specific solutions tuned for your business logic, user workflows, and compliance needs.
- Good AI outsourcing isn’t a black box. You get playbooks, docs, recorded walkthroughs, and internal onboarding support so your team owns what’s built.
- Long-term support without long-term bloat: AI isn’t “set it and forget it.” Outsourced teams offer tuning, retraining, scaling, and GTM support as your needs evolve—without ballooning your headcount.
If execution or stategy is the gap, this is your bridge. Most teams don’t fail because they lack ideas—they fail because they can’t get them shipped. With High Peak, you get an experienced AI expert who knows how to turn product vision into working software. Let’s talk about your AI execution plan—A 30-minute consult. No sales deck. Just clarity on how to move forward. |
Outsourcing AI strategy: How great partners lead from day one
A strong AI project starts before the first line of code. Here’s how elite AI outsourcing companies lead with AI strategy that delivers:
- Diagnose business needs and constraints: Not all AI is useful. A great partner will start with what matters: business goals, data realities, time pressure, and compliance boundaries.
- Frame a strategy tied to business outcomes: Your AI roadmap should connect directly to user experience, process improvement, or revenue—not generic “innovation” objectives.
- Map and prioritize AI opportunities: Which use cases are viable now? Which should wait? Great partners help you prioritize based on impact, readiness, and ROI potential.
- Create a build-and-ship roadmap: Phased plans that show what gets built, when, and how it connects to systems and users. Clear sprints, clear goals, no fluff.
- Identify risks before they become blockers: From data integrity to stakeholder pushback, great strategy includes a risk map—and a mitigation plan.
- Choose the right stack, tools, and partners: Build vs. buy, open-source vs. vendor—your partner helps you select architecture that scales and avoids technical debt.
- Enable internal teams through hands-on knowledge transfer: Every artifact—from architecture to training data—is documented, explained, and owned by your team. No vendor lock-in. No silos.
Plan your AI product the right way—from day one with High Peak. Whether you’re building your first AI feature or modernizing legacy workflows, it starts with clarity—not code. All you need is an AI consultation with our AI experts. |
The hiring trap: Why in-house AI teams fail early-stage or legacy orgs
It’s easy to assume that building an in-house AI team is the “mature” way to scale AI initiatives. But in reality, that path often slows companies down—especially if you’re a lean startup or a mid-sized enterprise navigating digital transformation.
Here’s why hiring internal AI teams often fails before it starts:
- Talent is scarce—and expensive. The best AI engineers are in short supply and command top-tier salaries, especially in North America, Europe, and APAC tech hubs.
- Ramp-up takes months. Recruiting, onboarding, and setting up the right infrastructure can drain critical time from fast-moving teams.
- R&D ≠ production. Internal teams often get stuck experimenting, but never ship. ML research alone doesn’t drive business outcomes.
- For startups: Every extra month of burn is a risk. Delayed shipping means missed traction, slower fundraising, and lost market share.
- For enterprises: The cost is higher, the velocity lower, and internal resistance (from security, IT, procurement) can stall progress entirely.
That’s why more companies are turning to AI outsourcing vs hiring internally. When you outsource AI development services to a trusted partner, you get execution-ready teams, proven infrastructure, and a clear path to deployment—without the hiring overhead.
Absolutely — here’s a refined and detailed expert version of the section that maintains SEO alignment (“outsourcing AI product development”) while offering deeper, clearer insights into what teams avoid by not rushing to hire prematurely.
This version is still skimmable (for AI Overview), logically sharp (for LLM ranking), and emotionally resonant (for your ICPs under pressure to scale fast but wisely).
What you avoid by outsourcing AI product development instead of hiring too early
Hiring AI talent too soon can backfire—especially when you’re still defining the problem, validating the use case, or building toward product-market fit. Here’s what you sidestep by choosing outsourcing for AI product development first:
- Months lost to recruiting, onboarding, and team ramp-up: Hiring AI engineers is time-intensive—especially in competitive tech hubs. Then come onboarding, tooling, data access, and alignment. That’s 2–4 months before you ship anything. Outsourcing gives you a team that starts executing in week one.
- Capital spent on infrastructure before validating need: It’s tempting to set up a full MLOps pipeline early—data lakes, vector stores, model registries. But until you prove the use case, most of that spend is speculative. Outsourced partners bring modular infra that grows only when it makes sense.
- Misalignment between AI research and real product value: Internal AI teams often focus on experimental models or overfitted accuracy metrics. Product outcomes—like speed, user clarity, or operational lift—can get sidelined. Outsourced teams are structured to ship usable, integrated features from the start.
- AI that never reaches your users: A working model isn’t a product. Without UX alignment, frontend integration, and feedback loops, many AI features die in notebooks. Outsourcing teams work across data, engineering, and design—so AI becomes part of the product, not an isolated experiment.
Getting locked into rigid, unscalable architectures: Early internal hires often over-engineer solutions or hardwire vendor choices that are hard to reverse. Outsourced AI development favors flexible, future-proof stacks—letting you adapt as the business scales or pivots.
What you actually need: AI outcomes, not headcount
The goal isn’t to hire AI engineers—it’s to deliver results your business can feel.
An AI outcome isn’t a model or a prototype. It’s something tangible:
- A manual process fully automated
- A complex workflow simplified for users
- Critical insights delivered at the moment they’re needed
It’s the kind of progress that shows up in faster ops, happier customers, or new revenue.
Example:
An industry-agnostic AI knowledge management software company had a backlog of unstructured PDFs—contracts, client checklists, and audit trails. Instead of hiring an AI team, they outsourced a purpose-built NLP, Generative AI, and LLM-backed file management solution. They deployed a production pipeline that auto-classified documents, extracted key entities, structured the unstructured raw data to actionable knowledge, built generative AI insights, and flagged risk conditions. What used to take 40 hours per client now takes less than 4.
That’s an AI outcome: measurable efficiency, faster onboarding, and a product users actually trust. No internal AI lab. Just traction that moves the business forward.
That’s what investors and execs want to see: traction, clarity, speed—not an internal AI lab. You don’t win by building headcount. You win by shipping outcomes.
Great AI isn’t about internal labs. It’s about faster workflows, clearer insights, and real product wins. If you’re ready to turn raw data into outcomes—without the overhead—we’ll help you scope, validate, and launch your first AI-powered feature in weeks. Book a demo and talk to our senior AI product strategist. |
Why AI outsourcing works in 2025
In 2025, outsourcing AI development services isn’t just a cost-saving move—it’s a strategic shortcut to market-ready AI features, without the delays and risks of building everything in-house.
For funded startups:
- Speed is survival. By outsourcing AI development, startups gain access to modular AI stacks and fractional teams that are ready to execute. There’s no need to recruit, onboard, or trial different approaches.
- MVPs are delivered in weeks—not quarters—because outsourcing partners already have the tools, workflows, and infrastructure in place. This removes early tech debt and helps founders focus on customer traction, not technical staffing.
- Sprint-based pricing models also keep costs predictable and aligned to outcomes. You only pay for shipped, usable value—not vague exploration.
For mid-sized enterprises
- Outsourcing allows teams to integrate AI into legacy systems without overhauling core infrastructure. It removes the need for large-scale hiring or bloated procurement cycles—both common blockers in enterprise innovation.
- More importantly, you can prove ROI in small, controlled pilots before scaling. That de-risks the entire roadmap and makes it easier to win internal buy-in from risk-averse stakeholders.
- The best AI outsourcing companies also bring governance, compliance knowledge (GDPR, HIPAA), and enterprise-grade design practices—so what you ship isn’t just technically viable, but fully operational from day one.
What to look for in an AI outsourcing company
Not all AI outsourcing partners are created equal. Choosing the wrong one means wasting time and budget on disconnected demos or over-engineered solutions that never ship. Here’s what high-performing teams look for when evaluating AI outsourcing companies:
Domain-specific experience: Look for a track record in your space—Fintech, Healthtech, SaaS, etc. Domain knowledge saves weeks of discovery and improves accuracy, compliance, and decision logic from the start.
A human-guided build process: Great partners don’t just apply prebuilt APIs. They work side-by-side with your team to understand user flows, constraints, and business KPIs—so the AI actually fits your product.
UX-first AI delivery: AI that isn’t usable doesn’t matter. Your partner should work across design, product, and engineering—ensuring that models are integrated into intuitive interfaces that create real impact.
Compliance and security awareness: If you’re in a regulated industry, your partner should already understand HIPAA, GDPR, SOC2, and audit trails. This isn’t optional—it’s foundational.
What you actually need: a fractional AI product team
Hiring a full AI team sounds impressive—but it’s rarely what startups or transformation-focused enterprises actually need. What you need is velocity without bloat. Precision without overhead. And most importantly, outcomes without long-term lock-in.
That’s where a fractional AI team comes in.
This model gives you the exact expertise you need—only when you need it. No long-term salaries, no drawn-out onboarding, and no over-committing before you’ve validated the use case.
Here’s how a fractional + modular AI product development approach works:
- Fractional strategy: Access strategic AI architects, ML engineers, and product-minded data scientists without full-time headcount. Teams ramp up in days, not quarters.
- Modular delivery: Need data wrangling for one workflow? Or model integration into an existing UI? You get discrete, composable delivery units—UX, infra, modeling, QA, observability—plugged in only when required.
- Repeatable product process: A strong partner follows a clear build process:
Align → Scope → Prototype → Launch → Scale
No ambiguity, no overbuilding—just iterative shipping that grows with your roadmap.
This is how real AI products get to market fast—without burning cycles on roles you don’t need yet.
How High Peak ships AI products
Outcomes, not overhead. That’s the principle behind every build at High Peak. We combine fractional AI teams with modular product delivery to move from idea to production in weeks—not months.
Step-by-step guide to building AI without a full team
- Step 1: Start with the user friction, not the model: What’s slowing users down, driving up costs, or hurting conversions? We begin with the real-world friction—not with theoretical ML research.
- Step 2: Map to the smallest useful AI task: We scope AI to what’s actionable: summarize, classify, extract, rank, generate, or cluster. Not every workflow needs a full LLM.
- Step 3: Build a vertical slice: One user flow, one model, one real UX. We ship something usable—not a backend-only sandbox. The goal is real impact from the start.
- Step 4: Use the leanest model that works: We avoid “over-modeling.” If OSS or hosted APIs solve it, great. If hybrid or fine-tuned models are needed, we evolve into it. The architecture grows with your traction.
- Step 5: Launch with tight observability: We track model confidence, latency, user behavior, and output trust. Why? Because feedback—not accuracy—is what powers product iteration.
Want to ship AI without the overhead? Choose High Peak
Build your first AI feature without hiring a team. Skip the recruiting delays and infrastructure setup. In your free strategy consult, we’ll explore your use case, assess technical feasibility. We’ll show how you can get to a working AI feature in weeks—not months—without sacrificing quality, speed, or control.