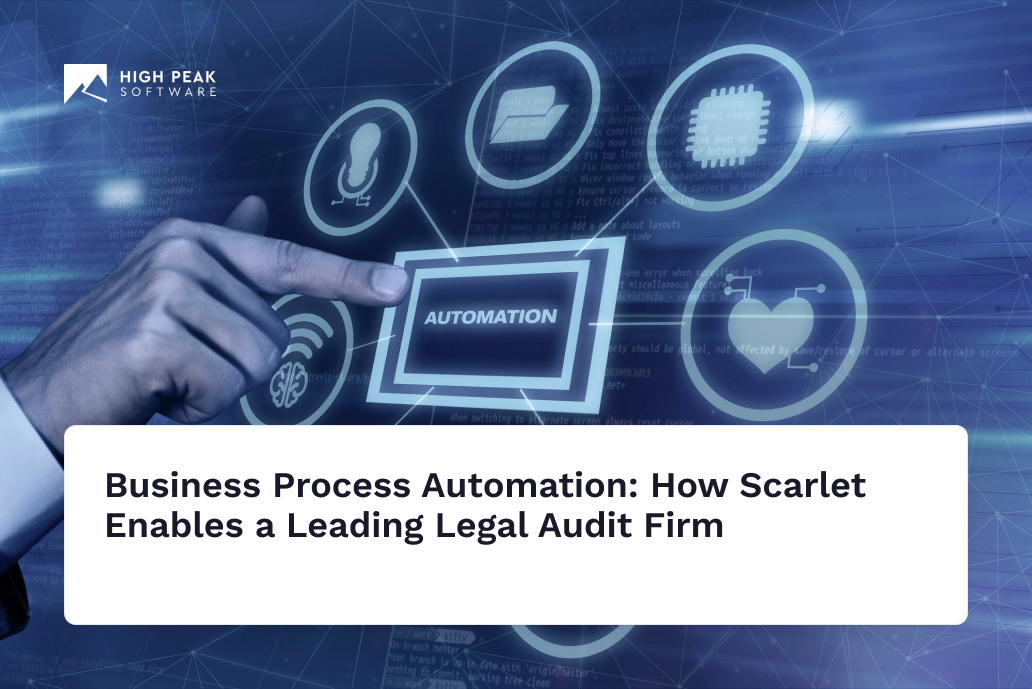
Table of Contents
Scarlet is an automated document processing system, developed by us. It is powered by intelligent machine-learning algorithms. It carries out a geometrical analysis of a document and accurately extracts relevant data for business process automation.
Scarlet is a customizable solution capable of processing any kind of document, including images and non-searchable PDF files, specifically tailored for your business requirements.
If you would like to know more about Scarlet, please visit the detailed product page.
Client Overview
Our client is an independent legal audit firm based in India that specializes in audit techniques aimed at helping companies achieve 100% compliance levels.
The organization provides various audit services, such as organization compliance reviews with respect to labour legislations, detecting non-compliances and potential liabilities, legal risk profiling, and other such services.
Problems
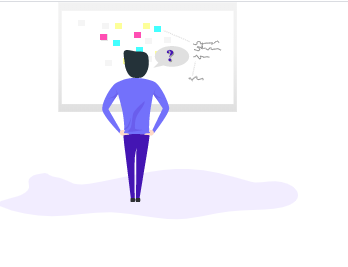
It is common knowledge that audit firms have to deal with mountains of paperwork, owing to several processes involved in the auditing process.
Our client, comprising 20 lawyers, currently performs monthly audits in various factories for its vendors. These vendors represent enterprise clients comprising tens of thousands of employees.
Depending on the number of employees or factory workers, the client picks out employee documents randomly and checks for any discrepancies. While this process may be feasible for organizations with fewer employees, conducting audit checks for a large organization with thousands of employees is nearly impossible.
This process of conducting manual audit checks further introduced problems, such as:
Unreliable processes — Because the auditing of heavy paperwork was done randomly, the process was not reliable or efficient. Many documents with discrepancies would go unchecked, thereby negatively affecting the laborers in the factories.
Non-sustainable methods — Manual classification and auditing of the documents across organizations was not scalable or sustainable in the long term. In addition, the entire process was not only time-consuming but also inefficient.
The client wanted to automate these manual processes and approached High Peak for the same.
Challenges
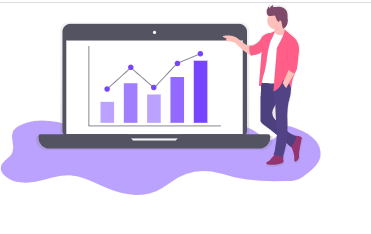
Some of the challenges faced by our client were:
Counterproductive Processes
Because the client conducted random audits at the factories, there was no way to ensure that all the documents with discrepancies were being audited and reprocessed.
Unreliable and Non-scalable Methods
The auditing process carried out by the client could not be carried out for every factory worker. Organizations allocate a significant amount of pension funds, otherwise known as PF, and other funds relative to the employee’s wage. By not accurately detecting discrepancies, the factory laborer would not be able to claim or receive adequate pension after retirement. Further, the client was limited by the amount of manual undertaking the team of advocates were able to handle, making this operation highly unscalable in the long run.
Manual Data Consumption
The client carried out processing and validation of data for all the documents manually. Because these documents are data heavy, processing and validating data was a highly error-prone and tedious process.
Data Accuracy
Because the audits were being carried out manually by the client, it was prone to human errors. Therefore, the team had to ensure that the system is capable of extracting data with the highest levels of accuracy.
Data Security
Our client was dealing with financial and workforce data, which is extremely sensitive. The team had to ensure that extraction of data from the documents was done in such a way that sensitive data was secure and tamper-proof.
Scarlet Enables Business Process Automation
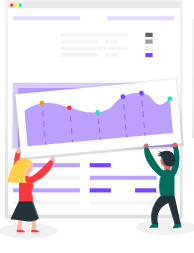
Scarlet was used to automatically in business process automation to classify three main documents–the Form T, EPF, and the ESI document.
Form T: This is a combined muster-roll and register of wages form which stores data related to employee attendance, overtime work, and monthly wage for every employee in an organization.
Employees’ Provident Fund (EPF): EPF is a monthly contribution made in the employees’ name from their monthly wage up until their retirement from the organization.
Employee State Insurance (ESI): The ESI is a contributory healthcare insurance fund that is self-financed by the employee and the employer as well.
Automated Document Processing for Structured and Unstructured Data
Scarlet first carries out a geometrical analysis of the document. This process analyzes the document and draws out the shape of the content in the document. For example, if the document contains a table, it would be identified as such by its even spacing, rows and columns, etc., whereas unstructured data would be identified by its free flow of text.
Each content or data in the document is associated with its attributes, such as font size, whether the text is bold, italicized, or indented, etc. Further, the system does a hierarchical mapping of all contents in the document, mapping parent data with child data, and so on, in the form of a tree.
This way, even unstructured data is given a specific structure and made configurable. The required output can be customized by setting certain rules for the data to be extracted. For example, if the user requires only the invoice number and the bill amount from an invoice document, the system extracts only those, leaving out other trivial data, and presents them as the final, structured output. Ultimately improving the overall business process automation.
Earlier the client was manually uploading each document in separate instances onto the system. With Scarlet’s built-in classifier all documents can be uploaded at once in a zip file format. Scarlet automatically classifies the documents, thereby increasing the processing speed and efficiency.
To achieve this entire process, the High Peak team implemented a complex combination of convolutional neural networks, recurrent neural networks, and segmentation to analyze and extract required data.
Accurate Data Extraction with an Improved OCR Pipeline
Scarlet can extract data from a document in three formats–tables, sections, and key-value pairs.
A key — value pair is a set of data items that are directly linked to one another.
For example, in a document, the term “Date of Joining” could be a key, and the actual date in a number format, say, “10/01/1995” could be its value.
Because every document has a different structure, the extraction of data depends on the type of document being processed.
Scarlet determines the various structures that are present in the document. Based on the structure, Google Vision is employed to extract the text data. This text data is pushed into a Deep Learning model, which is capable of predicting assigned key-value pairs, which are then extracted to be printed.
Because the Form T document contains multiple columns values such as payment of wages, minimum wage, maternity benefits, allowances, deductions, attendance data, and so on, Scarlet was used to perform table extraction to extract data from all columns in a tabular format. The extraction of data from such a document will be in the table format.
Further, data extraction for the ESI and EPF documents was done in a free-form text format.
The team employed the Google Vision OCR to scan a large number of documents in a given period of time, thereby improving the processing and data extraction speed.
Improved Data Validation
Scarlet enables the user to validate and update the extracted output in case the system fails to extract a part of the image.
High Peak developed a business rule engine to validate the data extracted from the documents. Scarlet first extracts the data and runs the extracted output on the business rule engine to check for missing values and other discrepancies.
For instance, if a particular column of a table from the Form T is missing from the extracted output, it can be rectified by selecting that column to be extracted. The final output will include the extracted column. Further, if the user wants to update textual contents on any of the selected columns or rows or sections, the updated contents are retained in the output.
In the case of the key-value pair extraction, the user can draw a bounding box in the document around the area that needs to be extracted. The system then extracts the information contained in this box, thereby reducing time and manual efforts.
If you would like to know more about Scarlet, please visit the detailed product page.
If you would like to know how Scarlet can enable your business process automation to mitigate overhead costs, please contact us now.