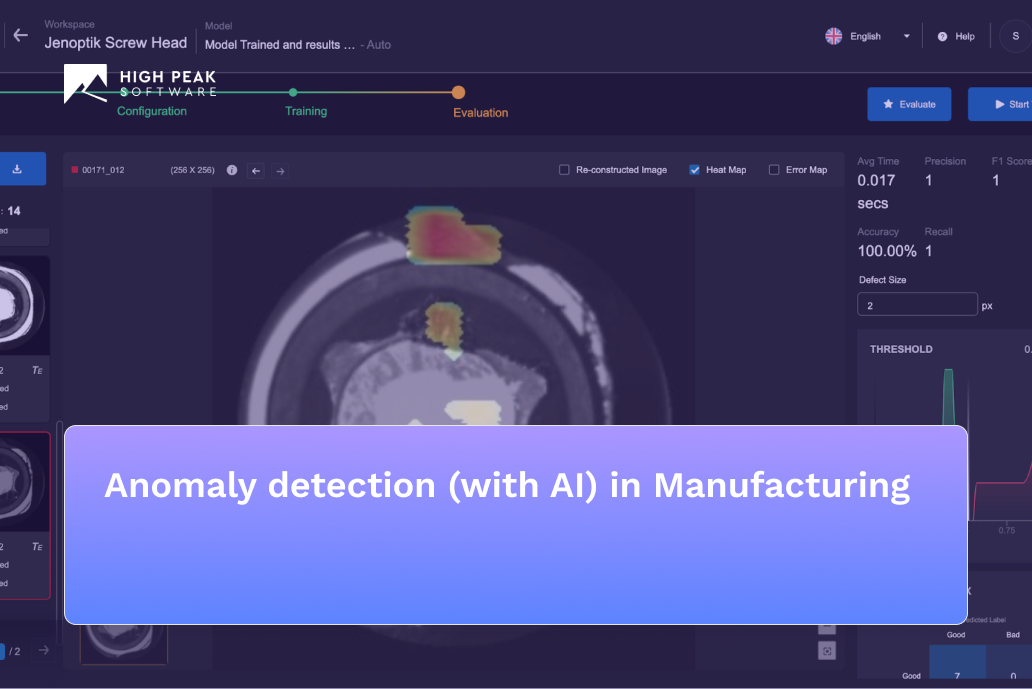
Table of Contents
- What is anomaly detection?
- Overall Equipment Effectiveness (OEE)
- Artificial intelligence at the machine level
- Types of machine learning/artificial intelligence techniques used
- Top features to consider in an automated anomaly detection software for the manufacturing industry
- Key use cases of artificial intelligence-based anomaly detection in the manufacturing industry
- Pros and cons of implementing AI in the manufacturing industry
- Leverage the best anomaly detection software built by High Peak
The market for anomaly detection is expected to expand significantly over the next few years, reaching a value of 5 billion dollars by 2026. This growth is driven by a steady increase of 7.5% each year. The onset of the Big Data technologies and the Internet of Things (IoT) have opened up unprecedented possibilities in the industrial ecosystem. Machines enabled with smart sensors constantly produce a large number of datasets. These datasets are processed to make better business decisions. This has led to an upsurge in Smart Manufacturing and the inception of industry 4.0.
To analyze machine data more efficiently and in real-time, identify rare occurrences, items, or events of concern. These rare occurrences have a diverging pattern from the majority of processed data. This can be achieved by using a technique called anomaly detection, a technology powered by artificial intelligence.
What is anomaly detection?
The data set defines an anomaly as a point or series of points that deviates from the normal behavior of the data. Anomaly detection is the act of detecting patterns of interest (outliers, exceptions, etc.). It is something that deviates from the expected behavior within the dataset(s). Anomaly detection has the ultimate goal of finding the outlier along with the value of insight the outlier provides.
Anomaly detection utility for industry 4.0
Today, modern businesses are leveraging data to drive key business decisions. With access to more data, it is vital to analyze and interpret the data correctly. As a result, finding the outliers is only the first step. It is because this is where the utility of anomaly detection comes in. Industry 4.0 has emerged as an ideal case scenario for amplifying ML and AI solutions to industrial processes, especially in monitoring and optimization.
With the inclusion of deep learning anomaly detection algorithms in various industrial processes, industries from different verticals are leveraging it for:
- Prevention of security breaches, threats, and cyber attacks
- Discovery of hidden performance opportunity
- Automated KPI analysis and reporting
- Better and faster call to action
In this article, we’re set to explore anomaly detection using machine learning and artificial intelligence in the manufacturing industry.
The manufacturing industry offers a great environment for the application of machine learning and artificial intelligence techniques. Until recently, major manufacturing data was only in use for troubleshooting purposes.
A single process has hundreds of parameters from sensors, if not thousands, so effectively determining the source of a problem in a process was arduous. With multiple processes set in place, it used to be hard to detect anomalies in time and often resulted in substantial loss of both cost and time.
Also, manufacturing facilities can leverage real-time data analysis for a competitive edge. This is enabled by AI-driven technologies and smart sensors, improving productivity, quality, and other key performance indicators (KPIs).
The manufacturing industry has coined Overall Equipment Effectiveness as one of its key performance indicators.
Overall Equipment Effectiveness (OEE)
The manufacturing industry landscape has changed significantly with the intervention of technology. Low-volume, highly personalized product work orders are replacing old, high-volume, non-personalized ones. With the market being highly demanding, extremely competitive at scale, hyperconnected and dynamic, it is a coherent need within the industry to improve and optimize all manufacturing processes. As a result, OEE emerged as one of the most important performance-measuring indicators in modern manufacturing facilities.
Also, manufacturing operations can effectively compare their utilization to its full potential through OEE, which serves as a benchmark indicator. It’s an industry standard for measuring manufacturing operation productivity. For example, a production line with an OEE score of 100% would produce goods with zero defects (quality), as fast as it can (performance), and with no downtime (availability). A 100% OEE is an ideal scenario that is not practically possible to achieve.
Organizations are leveraging AI-powered anomaly detection techniques for real-time condition-based monitoring of the industrial equipment in their production line to identify the most important areas for OEE improvement. In the majority of industry sectors, an Overall Equipment Effectiveness (OEE) score above 85% is typically recognized as a benchmark for excellence.
Artificial intelligence at the machine level
With the massive amount of data sets emerging via smart sensors—equipped at the machine level, the role of AI-driven, visual-based anomaly detection software becomes extremely critical. Also, they assist in processing and analyzing large data sets to provide meaningful insights for the business at scale, usually inconceivable by a human expert. Visual-based anomaly detection softwares, available in today’s market, help in many key aspects, such as:
- Providing real-time analysis on the data sets and sending an alert if it detects any unexpected pattern.
- Automatically process the data, modify the key parameters of generic patterns and identify the abnormalities in the data sets.
- Assist with end-to-end gap-free monitoring to identify the smallest of anomalies which otherwise go unnoticed by humans.
- Helps in filtering out signals from noise by decoding false negatives/positives produced by system thresholds.
- Empower business owners by providing predictive analysis on the captured data sets.
Types of machine learning/artificial intelligence techniques used
Machine learning-based systems and artificial intelligence solutions constitute the core of self-learning. Now, these systems are designed to learn on the go and deliver better solutions with every iteration. Datasets, collected by sensors, act as an input for these AI solutions, developing a data model over it.
A potential anomaly is raised each time there is an unusual pattern detected by these solutions and is cross-checked by a domain expert. Also, the system then takes the input from the domain expert, learns from it, and then builds upon the data model for future analysis. This keeps on iterating until the algorithm/solution reaches an acceptable level of accuracy.
These AI-driven solutions detect anomalies built on two types of learning techniques:
1. Supervised machine learning
It is the most commonly used technique as it is directed to a predefined target. This technique is used in use cases where we have input and output data, and we’re looking to map the function connecting the two. The aim of using this technique is to approximate the mapping function so that when a new input data is given, then the output variable could be predicted.
2. Unsupervised machine learning
This technique is suitable for cases where the output data is unknown. The aim of using unsupervised anomaly detection technique is to model the distribution in the given data sets to learn more about it.
Top features to consider in an automated anomaly detection software for the manufacturing industry
There are dozens of artificial intelligence-based anomaly detection solutions available in the market. Since manufacturing units are resource intensive , it becomes critical for stakeholders to integrate the optimal techstack in order to drive maximum output.
Below are the top 5 features one should consider while opting for an anomaly detection software for the manufacturing industry:
1. Real-Time Anomaly Detection
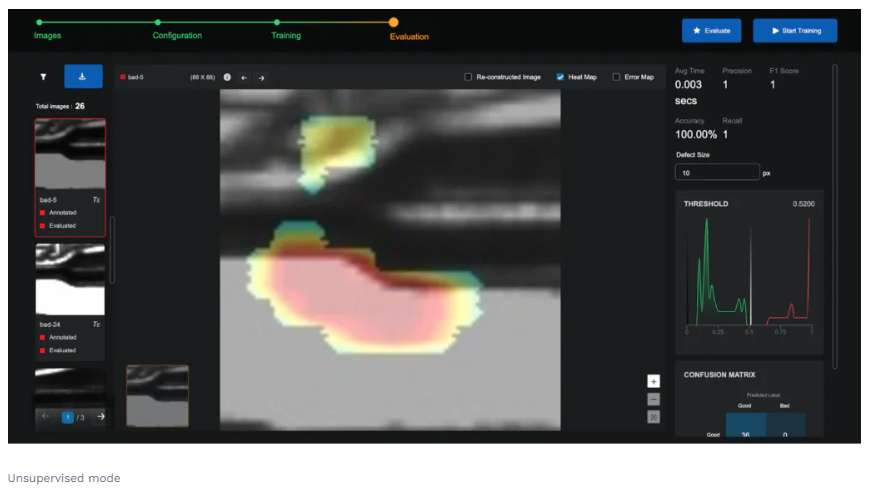
Today, businesses are heavily dependent on the value brought by their gathered data. To aid insights collected from the data, ML and analytics have become the trending tools. Since the domain requires real-time data to act upon and produce insights, an array of unwelcome data can create potential issues. Also, a quality AI-based platform should detect and highlight unexpected patterns in real-time to help businesses make better decisions.
2. Image Repository
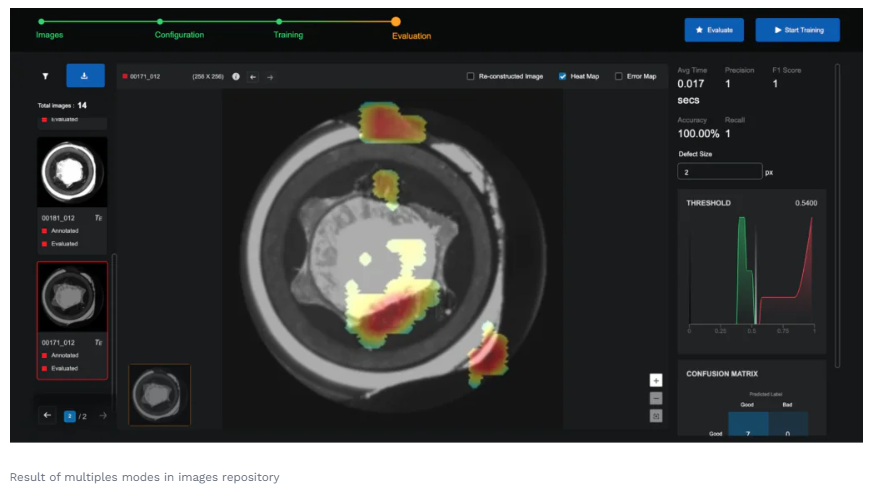
An anomaly detection software should capture, store, and label error-free as well as defective product images in the image repository. These labelled images can then assist the platform with training purposes when a new image is fed to the system.
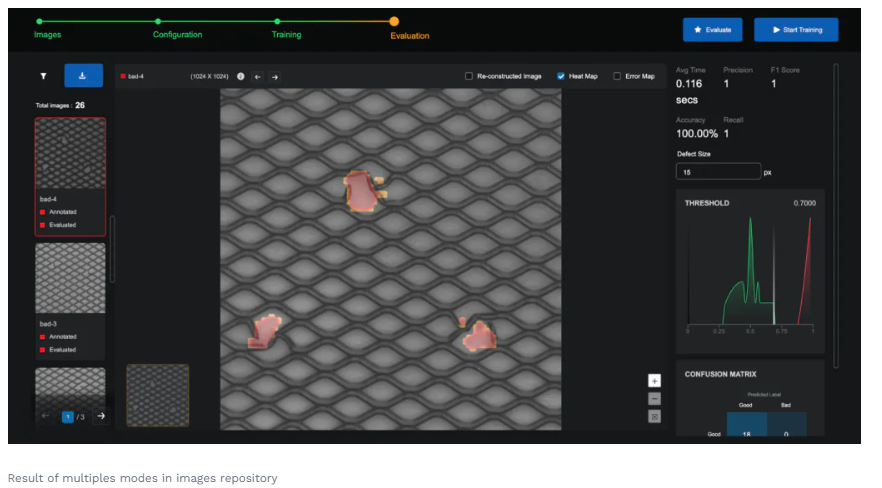
3. Model Repository
The model repository is the collection of all the models along with its results, that has been generated by the solution on the input data sets.
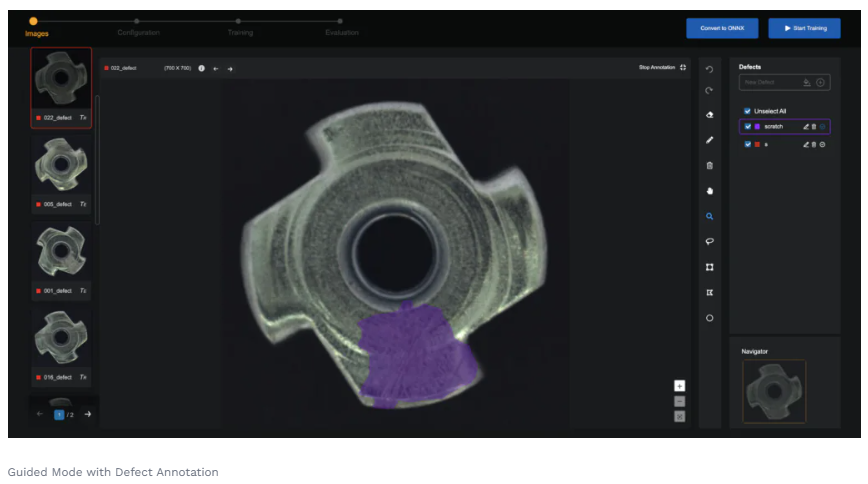
4. Automated Model Tuning and Training
Also known as hyperparameter tuning, provides the best version of the model by running multiple training jobs on an input dataset using the algorithm and ranges of hyperparameters provided to the solution.
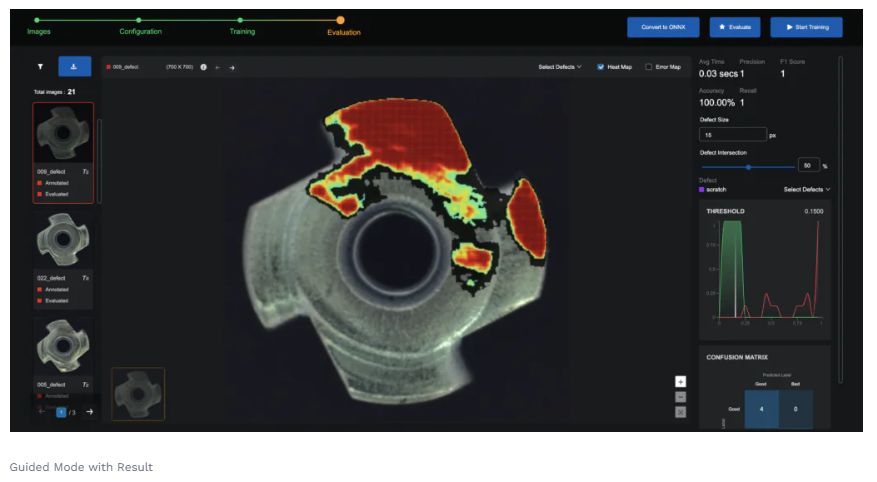
5. Model Validation
Model Validations is the set of processes and activities intended to verify that models perform as expected, in line with design objectives and business uses. It also helps in identifying the potential limitations & assumptions and assesses their possible impact.
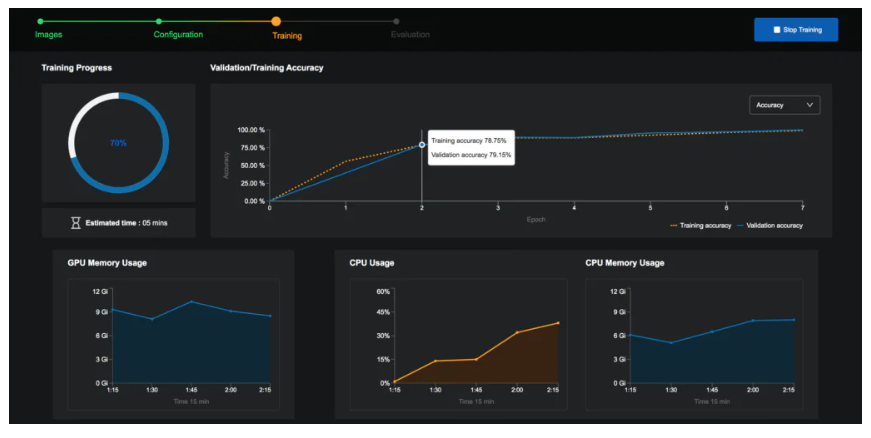
The above five features are must-to-have while considering a visual based surface anomaly detection software in the manufacturing industry. You can also check Vision AI; an automated anomaly detection software enabled with these features in action.
Key use cases of artificial intelligence-based anomaly detection in the manufacturing industry
Let’s take a look at two prominent real-life surface defect detection use cases in the manufacturing industry:
- General Electric has launched its Manufacturing Execution Systems (MES), powered by Predix (GE’s IoT platform), which uses sensors to capture and monitor every step of the manufacturing process automatically.
- The German conglomerate Siemens, has been using machine learning and neural networks to monitor its steel plants and improve equipment effectiveness for decades now. Also, with these solutions, Siemens aims to monitor, record, and analyze everything in manufacturing.
Pros and cons of implementing AI in the manufacturing industry
The introduction of AI and ML to the manufacturing industry represents a sea of change with many advantages and some challenges, opening doors to new business opportunities.
Some of the key pros of AI and ML in manufacturing include:
- Optimized production efficiency: Anomaly detection through AI algorithms greatly enhances the ability to identify bottlenecks within the manufacturing process. It streamlines operations for maximal throughput.
- Quality control enhancements: Implementing AI for anomaly detection in manufacturing ensures a high standard is maintained. It identifies defects before products leave the factory floor.
- Predictive maintenance proficiency: Anomaly detection via AI predicts when machines are likely to fail. Hence, maintenance can be performed preemptively, avoiding costly downtime.
- Supply chain optimization: With AI, anomaly detection refines supply chain operations. This leads to improved logistics and inventory management.
- Reduction in production costs: Anomaly detection helps in pinpointing inefficiencies and wasted resources. This ultimately trims production costs.
- Better energy utilization: AI systems with anomaly detection can identify power usage patterns that are wasteful. They optimize energy consumption across the manufacturing process.
- Adaptive manufacturing processes: AI-driven anomaly detection allows for swift adjustments. This adaptability meets fluctuating market demands and consumer preferences effectively.
- Enhanced risk management: Anomaly detection in manufacturing can foresee potential risks and mitigate them. This capability safeguards against operational disruptions.
Cons of AI and ML-based application in manufacturing are as follows:
- Acquisition of relevant data: This is a significant challenge. Quality, relevant data is the foundation of any AI/ML application. Without it, algorithm performance is hampered. Processing and cleaning the data to eliminate anomalies is a critical step that cannot be overlooked.
- The learning curve of ML algorithms: True. Machine learning models require time to learn from data before becoming sufficiently accurate. This learning process can consume substantial resources and time, especially initially or when adapting to new types of data.
- Interpretation of results: This is a notable issue. The results from AI/ML models can sometimes be non-intuitive, requiring expertise for correct interpretation. The ‘black box’ nature of some AI algorithms can result in diverse interpretations. This creates a gap in understanding decision-making processes, posing a challenge in transparency-requiring scenarios.
Leverage the best anomaly detection software built by High Peak
While organizations are using the latest AI/ML methods, tracking trends, and identifying opportunities and threats, the choice of automated anomaly detection software becomes extremely critical in gaining a competitive advantage.
Vision AI is an automated anomaly detection software built by High Peak. It is powered by intelligent machine-learning algorithms and computer vision. The platform carries out surface defect detection in products and is then represented on heat maps and error detection masks for easy defect representation.
Want to know more, contact us now!