Table of Contents
- Understanding the diverse types of AI models
- Benefits and challenges of AI business models
- Top 9 AI business models transforming industries
- How to navigate the AI business model landscape
- Challenges in securing AI and establishing ethical governance
- AI’s growing influence on business strategies
- Elements critical to new AI business models
- Selecting suitable AI business models for maximum impact
- The transformative power of AI on businesses
- Strategic planning for AI business models
- Defining the role and strategic value of AI business models
- Partner with High Peak and adopt top AI business models
Don’t you think Artificial intelligence is redefining the way we do business? As of 2024, 35% of businesses have integrated AI, underscoring the strategic importance of AI business models. This blog is dedicated to elucidating the prevalent AI business models and the various types of AI models that are capturing the attention of innovation leaders.
Our goal is to demystify these models within the current state of AI. Thus empowering businesses with the knowledge needed for informed decision-making in this evolving domain. Let’s start by showing you what happens behind the scenes when High Peak builds various AI development solutions for your business.
Understanding the diverse types of AI models
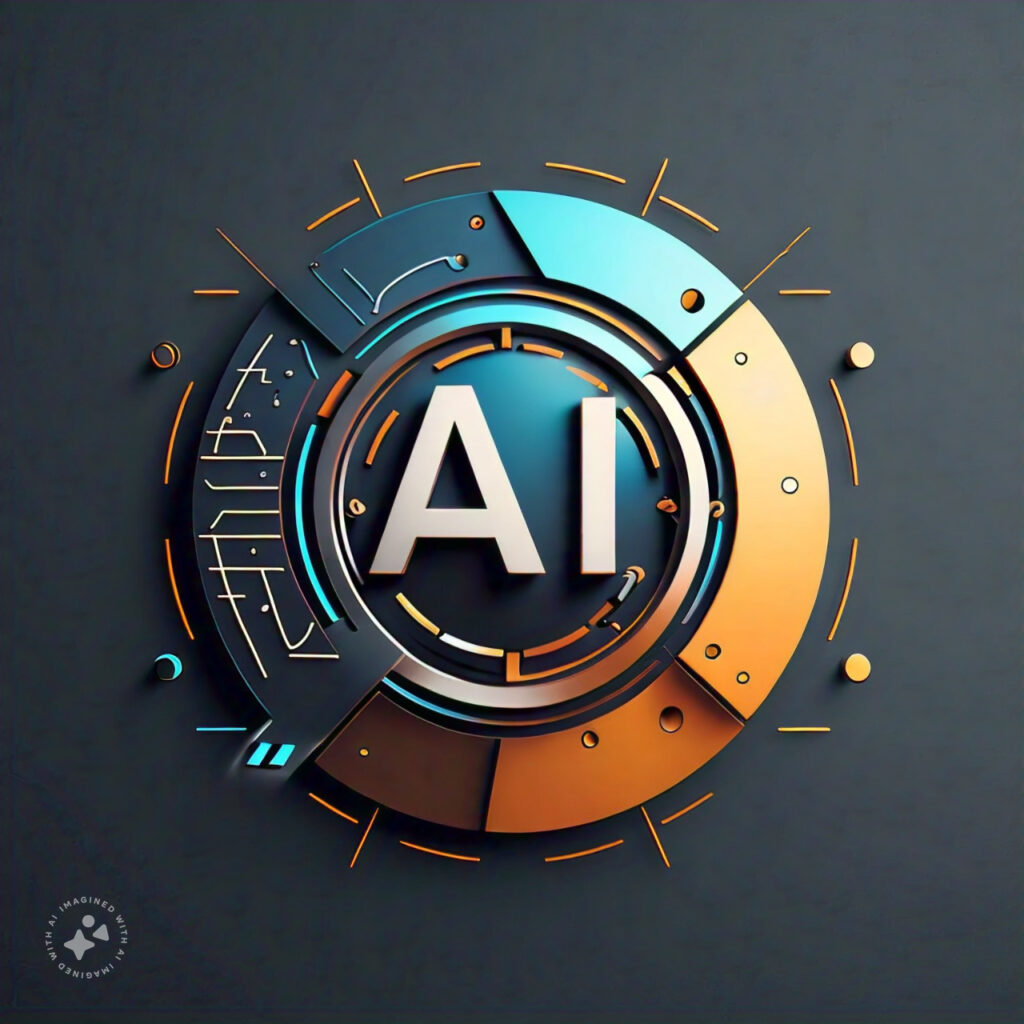
AI is transforming how we approach complex problems and tasks through diverse models. The various types of AI models enhance efficiency and insight, which are spearheaded by advancements from AI development companies. We’ll deepen your understanding of their contributions by examining AI methodologies, applications, and technologies. Here’s an in-depth guide to the different types of AI models:
1. Machine learning models are foundational
Machine learning is a pillar among types of AI models, enabling systems to learn from data and improve over time without explicit programming. Many AI development companies invest heavily in refining these models to make them more efficient and versatile.
- When to use real-life applications:: Utilized across various sectors such as healthcare for predictive diagnostics, finance for fraud detection, and e-commerce for personalized customer recommendations. Read this blog to learn more about AI in healthcare.
Supervised learning models guide AI with examples
- Linear regression: Offers predictions of continuous outcomes based on a linear relationship between input variables.
- Logistic regression: Excelling in binary classification tasks, determining the likelihood of inputs belonging to a specific category.
- Support vector machines: Finding a hyperplane that best separates classes, enhancing classification tasks.
- Decision trees and random forests: Simplifying decision-making processes, with Random Forests particularly shining, by managing overfitting to improve reliability and accuracy.
- When to use real-life applications: Employed in credit scoring, customer churn prediction, medical diagnosis, and more, where clear examples and outcomes guide AI learning.
Unsupervised learning models find patterns without guidance
- K-Means clustering: Efficiently groups data points by their feature similarities, informing various applications like customer segmentation.
- Hierarchical clustering: Forms a tree of clusters that elucidate data relationships at different levels of granularity.
- Principal component analysis: Crucial for dimensionality reduction, helping to highlight the most significant features in complex datasets.
- When to use real-life applications: Ideal for market segmentation, anomaly detection in network security, and organizing large data sets without predefined labels.
Semi-supervised and reinforcement learning models adapt dynamically
They bridge the gap between supervised and unsupervised learning, particularly useful when labeled data is sparse. AI development companies find these models invaluable for AI systems that require adaptability in unpredictable environments.
- Q-learning and DQN are prime examples of guiding AI agents via rewards to optimize decision-making in real-time scenarios.
- When to use real-life applications: Reinforcement learning models are pivotal in gaming AI, autonomous vehicles, and robotic control systems, where learning from interactions with the environment is crucial.
2. Deep learning models bring complexity and depth
These types of AI models feature layers that capture and represent data at various abstract levels, making them ideal for tasks requiring nuanced understanding.
- Convolutional Neural Networks: Outstanding for image and video analysis.
- Recurrent Neural Networks, LSTM, and GRU: Excel with sequential data and are vital for processing speech and text.
- Autoencoders: Used in feature learning and anomaly detection by reconstructing inputs from condensed information.
- GANs and Transformers, including BERT and GPT, are groundbreaking in generating robust and contextually rich text and images.
- When to use real-life applications: From facial recognition systems and autonomous drones to advanced language processing and creative content generation, these models underpin the next-gen AI applications.
3. Natural Language Processing models comprehend and generate language
AI development companies use these models to bridge human-computer interaction. The burgeoning field of NLP has various applications, from chatbots to sentiment analysis.
- Rule-based models: Employ crafted linguistic rules, often serving as the backbone for initial NLP apps.
- Statistical and Neural Network models: Leverage vast data to predict and interpret complex language patterns, facilitating advanced tasks like machine translation and contextual understanding.
- When to use real-life applications: Essential in developing intelligent assistant systems, content analysis tools, and real-time translation services, enhancing communication across diverse languages and contexts.
4. Computer Vision models process and interpret visual information
Employed in everything from autonomous cars to content moderation, these types of AI models help machines make sense of visual contexts.
- Image classification models: Distinguish image categories with remarkable accuracy.
- Object detection models: Pinpoint and identify objects in a broader scene, critical for applications like surveillance.
- Image segmentation models: Classify individual pixels to determine object boundaries, enhancing medical imaging and urban planning.
- When to use real-life applications: Fundamental in medical diagnosis through imaging, retail analytics through customer behavior monitoring, and enhanced safety in autonomous driving systems.
5. Predictive models forecast future trends
These models extrapolate future data trends from historical inputs, making them indispensable for financial forecasting, resource allocation, and market analysis.
- Time series forecasting models: Offer powerful tools for predicting future occurrences due to their advanced algorithms.
- Predictive LSTM networks: Similarly equip analysts and businesses with the capability to forecast trends with enhanced accuracy.
- When to use real-life applications: Critical in stock market analysis, weather forecasting, and supply chain optimization, enabling proactive decision-making based on predictive insights.
6. Hybrid models amplify AI capabilities
Integrating various types of AI models and data sources, these models are refined by AI development companies for superior accuracy and functionality.
- Ensemble models: These leverage the collective intelligence of multiple models to boost prediction accuracy.
- Multi-modal models: These decrypt complex sets of heterogeneous data, enabling richer analysis and interpretation.
- When to use real-life applications: These are useful in complex decision-making scenarios like precision medicine and financial risk assessment, where insights from diverse data forms are crucial for accurate outcomes.
7. Specialized AI models focus on niche applications
Evolving from general applications to commendable specifics, these types of AI models are designed to excel at distinct tasks.
- Recommendation systems: These enhance user experience by intuitively predicting user preferences.
- Anomaly detection models: These play a vital role in preventive maintenance and fraud detection.
- Optimization models: These streamline processes and solve complex optimization problems, highlighting their utility in logistics and network configurations.
- When to use real-life applications: From powering personalized content streams on platforms like Netflix to detecting unusual transactions for banking security, these models tailor AI’s benefits to specific industry needs.
Benefits and challenges of AI business models
AI business models are revolutionizing industries by offering unprecedented efficiency, decision-making capabilities, and customer experiences. However, these advances come with a set of challenges, including high implementation costs, ethical concerns, and data dependency. Understanding the benefits and obstacles of these models is crucial for businesses aiming to leverage AI effectively. Let’s see them in detail:
Benefits of AI business models
Enhanced efficiency and productivity: AI business models automate routines and optimize workflows, allowing teams to focus on strategic tasks. This improves overall efficiency and productivity.
- Improved decision-making capabilities: These models analyze vast datasets to offer quick, accurate insights, enabling data-driven decisions that outpace traditional methods.
- Scalability of services: AI facilitates service scalability, allowing businesses to adapt quickly to changes without a corresponding increase in costs or manpower.
- Enhanced customer experiences: AI predicts customer needs and provides personalized solutions, significantly improving satisfaction and loyalty.
- New revenue streams: AI business models can unlock innovative revenue avenues, leading to market differentiation and a competitive edge.
Challenges of AI business models
- High initial investment and implementation costs: Significant investment is needed for AI implementation, including technology procurement and skilled staff hiring from AI development companies.
- Ethical and privacy concerns: Types of AI models must be managed to avoid ethical and privacy breaches, which can severely impact consumer trust and regulatory compliance.
- Dependence on data quality: The success of AI business models depends heavily on high-quality data; poor data can misinform decisions and lead to setbacks.
Top 9 AI business models transforming industries
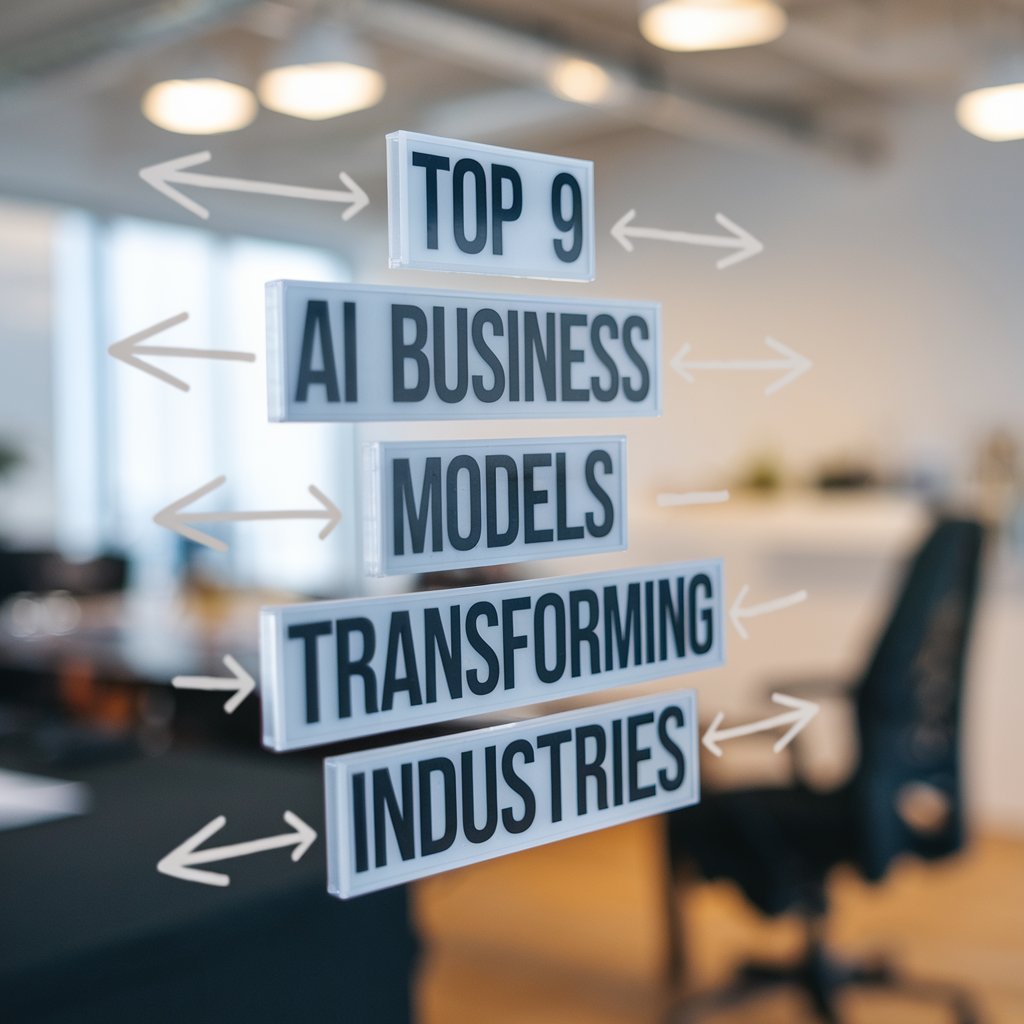
AI business models are driving a transformative wave across multiple industries. By harnessing the power of artificial intelligence, companies are not only redefining efficiency and value creation but also reshaping their growth strategies and revenue streams. Here’s a look at the top AI business models that are steering this change.
1. AI as a service (AIaaS) and subscription models: Blending services and recurring revenue
AI as a Service (AIaaS) is a popular business model that enables companies to leverage AI without investing heavily in the underlying technology. AIaaS providers deliver AI-driven capabilities through a cloud platform, offering them on a subscription basis. This model ensures recurring revenue for providers while offering flexibility to businesses seeking AI capabilities without long-term commitment.
2. Outcome-based pricing and value creation
Outcome-based pricing in AI business models focuses on the value delivered rather than the features provided. For clients, this means payments are directly tied to the performance and outcomes of the AI solution. This model is significant for companies that prioritize results, as it aligns incentives between the solution provider and the client, encouraging constant improvement and fine-tuning of AI applications.
3. Data monetization strategies in AI
Data monetization involves using data as a strategic asset to generate revenue. In AI business models, data is not just fueling AI algorithms but is also a valuable commodity. Businesses are capitalizing on anonymized datasets, selling insights or analytics services powered by AI to other companies. This model turns data into a profit center rather than a cost center.
4. Freemium and premium models: Balancing access and premium features
AI development companies offer freemium models to provide basic AI functionalities for free while charging for advanced features. This strategy is designed to entice users with accessible services and then encourage them to upgrade to more sophisticated, premium offerings. It allows users to experience the value of AI firsthand, increasing the likelihood of them investing in expanded capabilities.
5. AI-integrated products: Enhancing traditional offerings
Adding AI capabilities to existing products can significantly increase their value and utility. For instance, smart appliances that can predict their own maintenance needs or software with intelligent recommendation engines lead to greater user engagement and satisfaction. AI business models that incorporate AI into traditional offerings often find a competitive edge in their markets.
6. Platform-based models: Building scalable solutions
Platform-based AI business models serve as springboards for scaling services. These platforms are ecosystems where third-party developers can create and share their own AI-driven applications. This model thrives on network effects, where the value of the platform increases with the number of users and applications it supports.
7. Consulting and custom AI solutions: Tailored expertise and innovation
Custom AI solutions are at the heart of consulting-based AI business models. These models rely on the expertise and innovative capacity within AI development companies to build personalized AI systems for businesses. With these tailored solutions, clients receive AI applications that are perfectly aligned with their unique operational needs and strategic goals.
8. Ecosystem collaboration and extended enterprises
Ecosystem collaboration among AI business models is key to amplifying value. When different entities, such as AI developers, data providers, and hardware manufacturers, work cohesively, they create an extended enterprise that collectively drives forward innovation. This interconnected approach leverages shared expertise and cross-sector insights to produce more sophisticated AI solutions.
9. Consulting services: Assisting businesses in AI adoption
As adopting AI becomes imperative for staying competitive, many businesses seek guidance on how to integrate these technologies into their operations. Consulting services in AI business models provide this guidance, helping companies understand the types of AI models that best suit their needs, navigate implementation challenges, and harness AI’s full potential for transformational success.
How to navigate the AI business model landscape
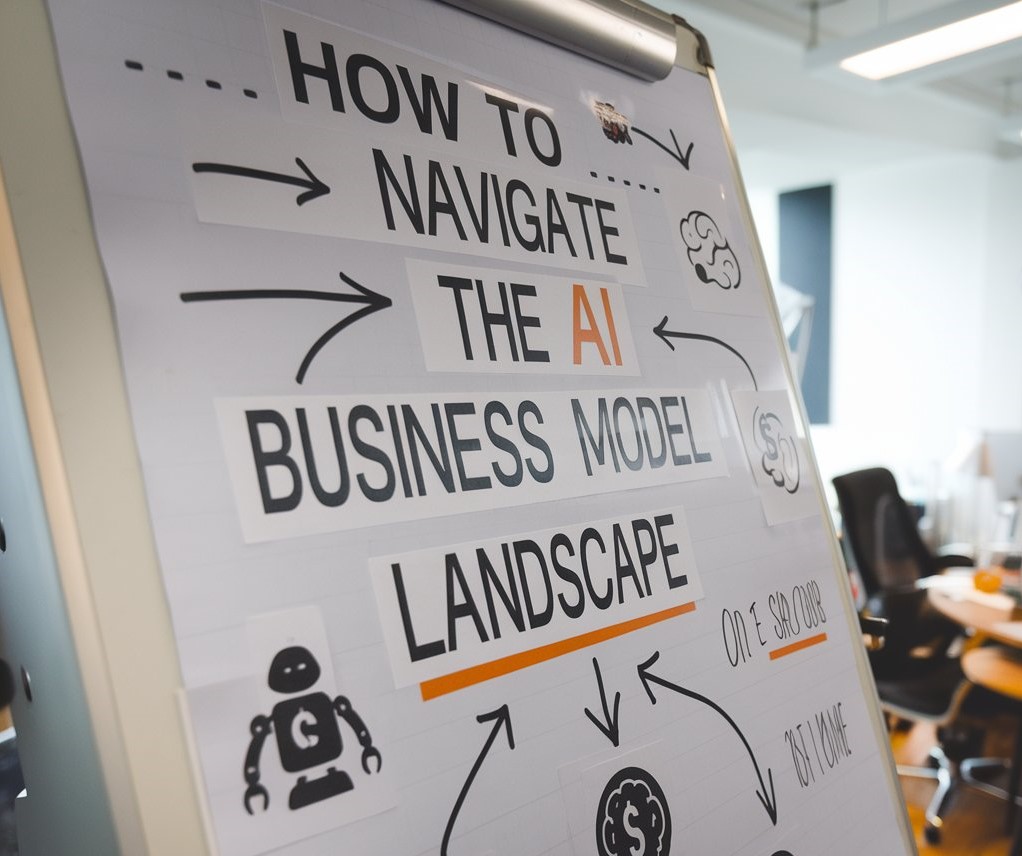
Understanding AI business models is essential for businesses that want to successfully adopt artificial intelligence. Making the right choices in AI business models can ensure that a business integrates AI in a way that aligns with its specific needs and goals.
Choose between open-source and commercial AI options
Deciding between open-source and commercial AI options is a fundamental step in navigating AI business models. Companies need to consider customization and cost savings offered by open source. At the same time, they should also evaluate commercial AI options for their higher support and enhanced security. Each company must make a choice that fits its capabilities and objectives.
Deciding between cloud-hosted and private AI
Another consideration within AI business models is deciding on the hosting environment. Should the AI be cloud-hosted or on-premises? A cloud-hosted AI can be scalable and cost-effective. However, a privately hosted AI offers more data control. Companies face this choice, focusing on their scalability needs, data security concerns, and the level of control they wish to maintain.
Understand and manage data for AI
For all types of AI models, data is paramount. A deep understanding of data requirements is part of effective AI business models. Companies need to assess data quality, ensure sufficient volume, and meet compliance standards. They need to process and store this data securely to fully utilize the potential of AI.
Develop a comprehensive AI policy
Developing a strong AI policy is an integral element of AI business models. It should address data use, model training, and the transparency of AI operations within the company. A solid policy is vital for ensuring ethical AI use and clarifying the roles and responsibilities of all employees.
Challenges in securing AI and establishing ethical governance
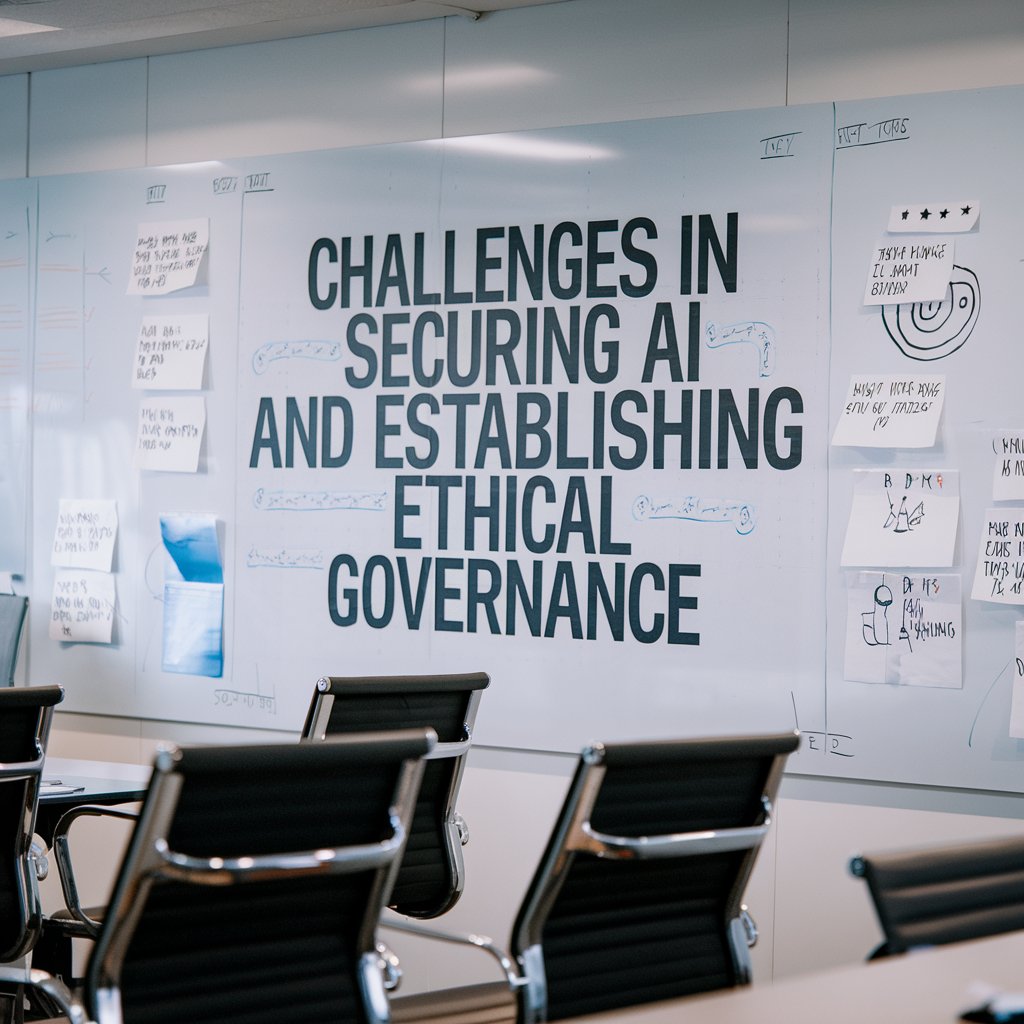
In AI business models, the push towards securing AI systems and establishing ethical governance is paramount. This section examines various facets involved in minimizing risks and setting up a robust framework for AI applications.
Reducing risks associated with AI
To safeguard AI business models, it’s imperative to minimize risks. This demands thorough risk assessments and preventive strategies tailored to AI’s unique vulnerabilities.
Crafting robust governance for AI systems
Establishing strict governance frameworks is essential. These frameworks should guide AI business models, ensuring they adhere to ethical and legal standards.
Commitment to ethical AI
Each AI initiative should incorporate ethical practices as its core principle. This involves creating and enforcing policies that reflect fairness and social responsibility.
Promoting transparency and responsibility in AI
AI business models must be transparent and accountable to build trust. Clear communication about how AI systems operate and the decisions they make is a key factor in gaining stakeholder confidence.
AI’s growing influence on business strategies
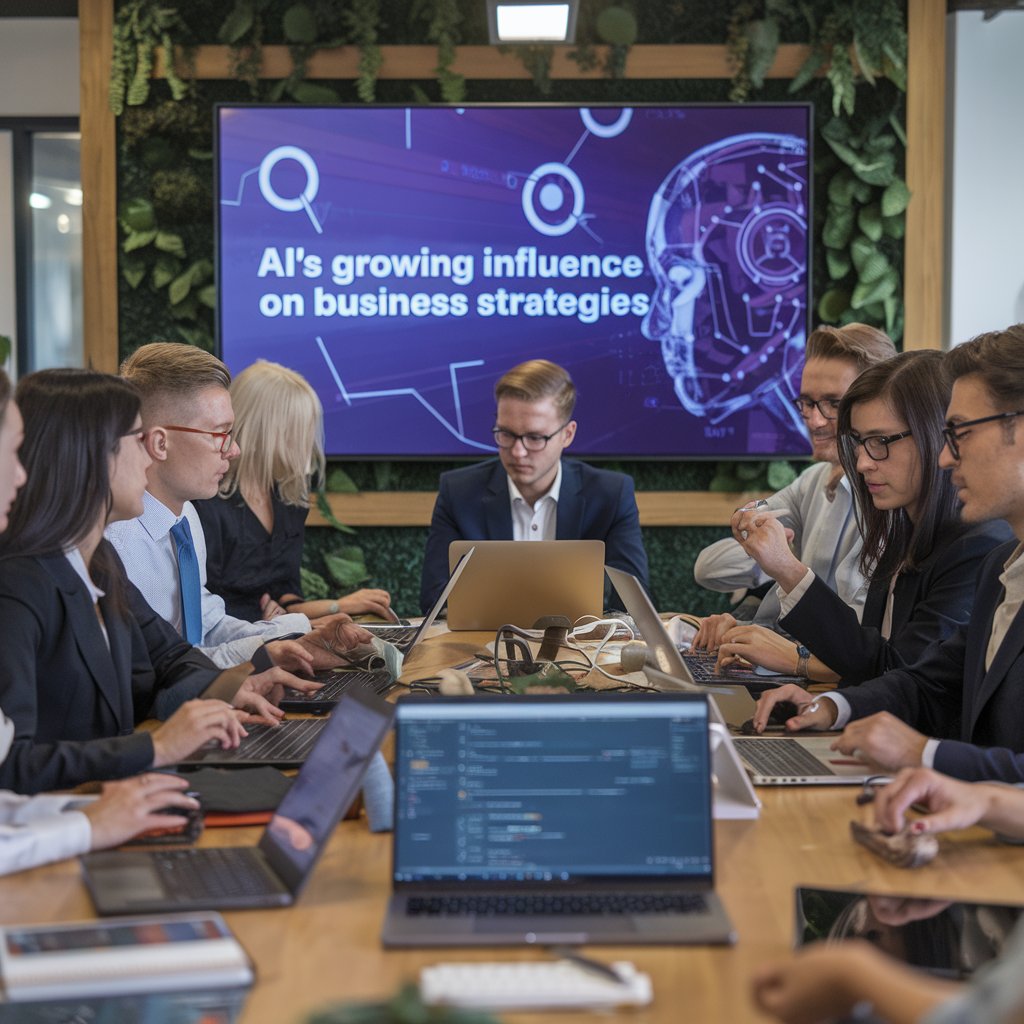
The influence of AI on business strategies can’t be understated, with AI business models acting as a key driver in revolutionizing industries. Here, we delve into how adaptive feedback, globalization of technology, and collaboration are shaping the future.
Integrating feedback to improve AI systems
Competent AI business models continuously refine their performance by using feedback. Such loops are essential to ensure relevance and efficiency.
Tailoring AI solutions to address local issues with global technology
Successful AI business models often balance universal applicability with localized customization. They solve regional issues by adapting to global AI developments.
Fostering collaboration in AI initiatives
Cooperative efforts, including open-source endeavors, are accelerating innovation in AI development. Collaboration is a cornerstone of many successful AI business models.
Pioneering new paths in AI with a focus on agility and innovation
AI development companies leading the market often excel at breaking new ground. They adapt swiftly to change, ensuring their AI business models stay at the forefront.
Creating personalized experiences through AI
AI business models that prioritize customized user experiences set the stage for enhanced customer loyalty and satisfaction.
Elements critical to new AI business models
Understanding what fuels the growth and sustainability of AI business models is key to leveraging this technology effectively. This section discusses the primary drivers and value propositions that underpin successful AI initiatives in the modern business landscape.
Key growth drivers shaping AI business models
Recognizing and leveraging the growth drivers within the AI sector is vital for the success of AI business models. This includes technological advancements and consumer demand.
Delivering value through AI
AI business models are distinguished by their capacity to create and deliver unmatched value. They revamp traditional services or introduce revolutionary products.
Utilizing AI’s predictive insights for strategic advantage
The ability of AI to anticipate future trends gives businesses a strategic edge. AI business models that harness this predictive power can devise more informed strategies.
Selecting suitable AI business models for maximum impact
The selection process of AI business models plays a crucial role in determining their impact and success. This segment explores key considerations, including market analysis, customer understanding, and competitive assessment, essential for making informed choices.
Analyzing market and revenue potential for AI business models
Choosing the right AI business models depends on a clear understanding of the target market and expected revenue streams.
Identifying your prospective customer base
AI business models vary, and so do customer expectations. Understanding your audience ensures that the chosen AI model aligns with their needs.
Assessing resources and the competitive landscape for AI
Comprehensively evaluating the resources at hand, the cost of implementing AI, and the existing competition is crucial. It helps determine the sustainability and competitiveness of AI business models.
The transformative power of AI on businesses
AI is fundamentally transforming the way businesses operate, with AI business models leading the charge towards greater innovation and efficiency. In this section, we look at how AI is reshaping business processes and the essential role of strategic planning.
Assessing the true impact of AI on business growth
AI has proved to be a driving force in optimizing business operations and spurring innovation. Companies leveraging AI business models see tangible increases in efficiency and expansion.
Seamless business process integration via AI
AI business models allow for the fluid integration of different business segments. This results in a unified and efficient operational structure.
Strategic planning for AI business models
Strategic planning is crucial for the successful integration of AI into business practices. Here, we navigate the intricacies of adapting to change, evaluating AI models, and understanding the strategic value and deployment of AI business models.
Adapting to change: A necessity for AI integration in business
Successful AI business models require a willingness to adapt. Businesses must evolve with technological trends and market demands to remain competitive.
Evaluating different types of AI models for strategic planning
Comparing various types of AI models reveals insights for strategic planning. It allows businesses to tailor their AI strategies to align with organizational goals.
Defining the role and strategic value of AI business models
The distinction between AI tools and comprehensive AI business strategies is critical for realizing strategic value. This final segment underscores the importance of understanding and strategically applying AI business models for optimal advantage and value creation.
Distinguishing between AI tools and AI business strategies
There’s a need to differentiate types of AI models from AI business models. While AI models refer to the technical aspects, AI business models pertain to their strategic use for value creation.
Integrating AI into business for strategic enhancement
Successful AI business models involve the strategic application of AI. This improves operations and should be an ongoing alignment of AI capabilities with business objectives.
Partner with High Peak and adopt top AI business models
As we explore the top AI business models of 2024, the importance of AI services cannot be overstated. Partnering with a custom AI development company like High Peak is pivotal for businesses aiming to stay ahead.
We offer tailored and custom AI solutions, empowering organizations to harness the full potential of AI technologies.
Don’t wait! Book a consultation with High Peak and ensure your competitive edge in tomorrow’s dynamic market.